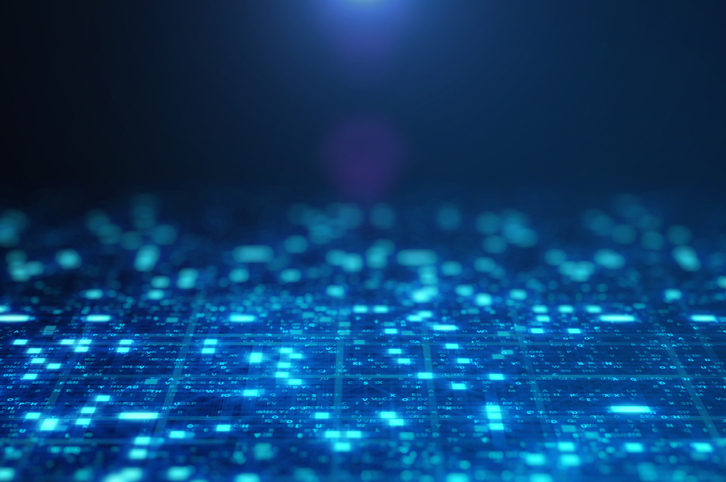
Many businesses struggle with demand forecasting. Whether you run a small business or a large enterprise, the challenge of predicting customer behavior and stock levels never gets easier. Even major organizations like Target and Walmart that are able to afford teams of data scientists have recently reported struggles with excess inventory due to poor demand forecasting.
During this time of global uncertainty, many businesses have adopted a just-in-case mindset. They’ve relied on archaic methods of forecasting, scouring old data and drawing poor conclusions based on past problems.
But understanding demand accurately shouldn’t be so much of a struggle in 2023. Even as we battle post-pandemic turmoil, we now have clear alternatives to legacy forecasting tools — thanks to artificial intelligence (AI). And we don’t need endless reams of historical data to access the real-time patterns necessary to accurately forecast demand. In fact, AI-driven demand sensing has been shown to reduce inventory errors in supply chain management by up to 50%, according to McKinsey & Co.
Every company produces data, of course, but it’s almost all trapped in siloes and walled-point solutions that have evolved for specific tasks over many decades. Siloes emerge for noble reasons — they represent a business’s attempts to organize and become structured.
Truthfully, siloes are useful in many scenarios, but if the boundaries between them are too sturdy and there’s a lack of effective communication, siloes will negatively impact business, putting more pressure on processes. Inaccuracies are most common in silo-heavy organizations because teams and departments just don’t have enough of a shared language. Rigid siloes also make data, even good data, less credible.
When working with ThroughPut’s clients, I’ve seen AI make all the difference in demand forecasting. That’s because it can pull from disparate datasets, using real-time patterns to sense the demand around the corner rather than just assuming future demand from past events.
Using an AI-driven system will pick out time-stamped data — regardless of barriers — and rapidly stitch together a global vision of your virtual supply chain network. Supply chain AI processes the best signals from the noise that is constantly being generated by your disparate data systems and turns the din into a song you can understand.
Furthermore, AI is superior at analyzing and making sense of data in vast quantities; yet it also doesn’t need much information to learn. AI trained for real-world applications already intuits which data signals to extract from an ocean of noise, so it can solve needs before they cause problems.
The quality of data is most important, not the quantity, and delaying the use of AI to sense demand is only going to cause current supply challenges to stagnate and potentially get worse. From there, share prices and shareholders suffer. We are seeing this today across industries: innovation laggards and slow adopters paying the price for relying on old forecasting methods.